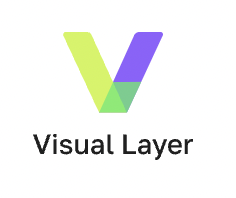
Visual Layer provides better visual data infrastructure to help gain insights, store, maintain and curate large image repositories and their metadata.
Visual data (images, videos, and other sensor data) has grown tremendously in recent years, with impactful applications in areas like social media, self-driving vehicles and manufacturing. Although much investment has been made in labeling platforms, the management, curation and validation of visual data is usually done with home-built tools that lack robustness and the ability to scale. This lack of tools leads to low data quality and wasteful data handling practices, hindering AI model quality and development agility.
At Visual Layer, our hypothesis is that there is strong demand for management tools for visual data. To validate this hypothesis, we created our free tool fastdup, a popular free tool providing basic, but highly scalable, building blocks for visual data. e.g., finding duplicates, identifying outliers, clustering, search, image statistics and detection of bad labels. In 6 months, fastdup was installed more than 145,000 times and gained significant community traction. fastdup has been already deployed in production by multiple Fortune 500 customers.
While fastdup is a free profiler that points issues in user's visual data, Visual Layer is building an enterprise cloud version which can automatically curate and fix error in the visual data. Our enterprise platform connects to cloud-based raw data storage services on one end, and AI/computer vision development tools on the other. Visual Layer includes three components: visual data management tools, deep learning-based data understanding, and automated data visualizations. With Visual Layer, computer vision engineers/ researchers can focus on building high-quality models and gaining data insights, while our platform manages, stores, maintains, cleans and curates their large image repositories.
There are multiple benefits for using visual layer. It frees computer vision team to deal with algorithms and not with data cleaning (a gory task no one likes to do), speeds significantly the model building cycle, significant savings on annotation costs, reduced storage, compute and network costs and improves the resulting models.